Predicting Peaks in COVID-19 Cases
Online search data can help inform the public health response to COVID-19
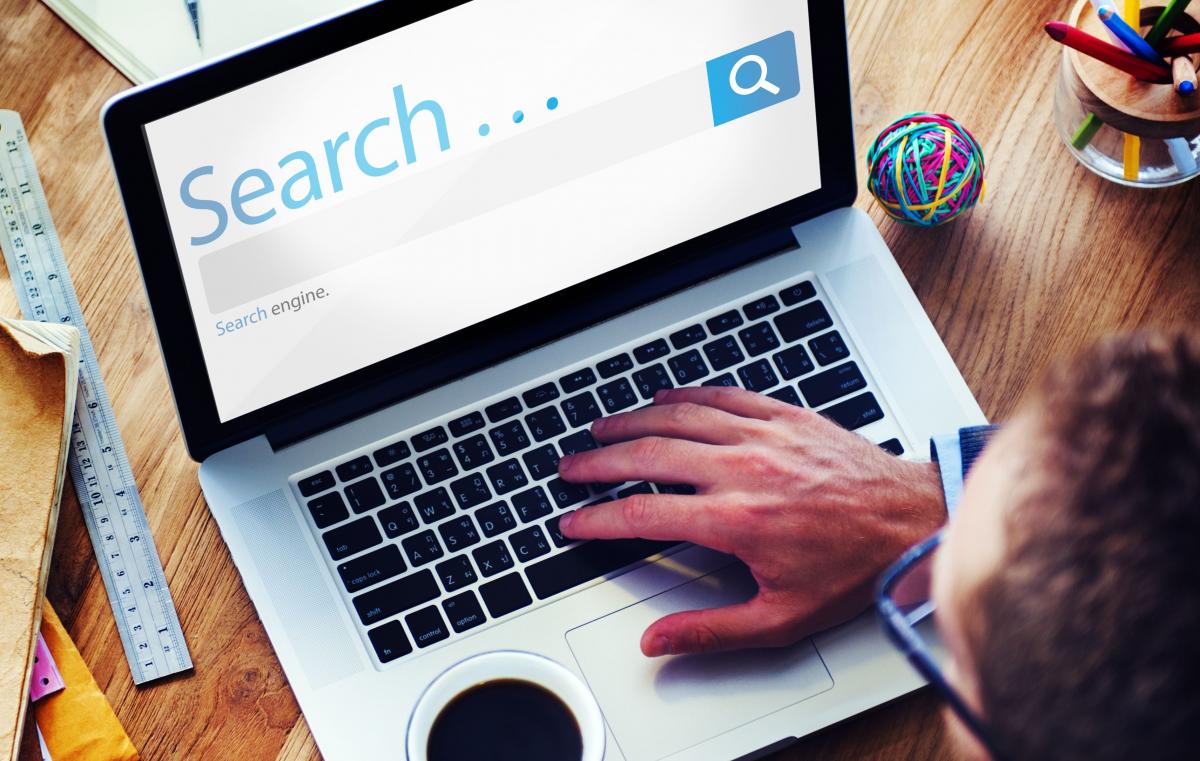
Online search data can help inform the public health response to COVID-19
Analysing internet search activity is an established method of tracking and understanding infectious diseases, and is currently used to monitor seasonal flu. The new findings show that online search data can be used with more established approaches to develop public health surveillance methods for novel infectious diseases as well.
For the paper, published in Nature Digital Medicine, researchers used COVID-19’s symptom profile from existing epidemiological reports to develop models of its prevalence by looking at symptom-related searches through Google.
They then recalibrated these models to reduce public interest bias – that is, the effect media coverage has on online searches. This enabled them to predict a peak in cases when applied to COVID-19.
Academics working on the models have been sharing their findings with Public Health England (PHE) on a weekly basis to support the response to the disease.
Lead author Dr. Vasileios Lampos (UCL Computer Science) explained: “Adding to previous research that has showcased the utility of online search activity in modelling infectious diseases such as influenza (e.g. https://fludetector.cs.ucl.ac.uk), this study provides a new set of tools that can be used to track COVID-19.
“We have shown that our approach works on different countries irrespective of cultural, socioeconomic and climate differences. Our analysis was also among the first to find an association between COVID-19 incidence and searches about the symptoms of loss of sense of smell and skin rash. We are delighted that public health organisations such as PHE have also recognised the utility of these novel and non-traditional approaches to epidemiology.”
Academics developed the uncalibrated model by choosing search terms relating to COVID-19 symptoms, identified by the NHS and PHE. The terms were weighted according to their ratio of occurrence in confirmed COVID-19 cases.
This model provided useful insights including early warnings and showcased the effects of physical distancing measures.
The calibrated version, which took news coverage into account, enabled academics to provide PHE with a model to more accurately predict surges in the UK.
The model was applied in several countries, including the UK, USA, Italy, Australia and South Africa, among others. They found that the same pattern appeared, in that surges in cases were predicted by their model.
Co-author Michael Edelstein, Professor, Population Health, Azrieli Faculty of Medicine of Bar-Ilan University, said: “Our best chance of tackling health emergencies such as the COVID-19 pandemic is to detect them early in order to act early. Using innovative approaches to disease detection such as analysing internet search activity to complement established approaches is the best way to identify outbreaks early.”
The team is confident that these non-traditional data sets and methodologies will continue to be integrated in conventional epidemiological systems, and always in a privacy-preserving manner.
“We can at least use the plethora of data sets around COVID-19 for further experimentation and validation of such techniques in an attempt to complement current epidemiological approaches and be better prepared for the next pandemic,” Dr Lampos added.
The research was supported by various funding bodies and organisations including the EPSRC, MRC/NIHR and Google Health.
PHE’s most recent report using the calibrated model is available here.